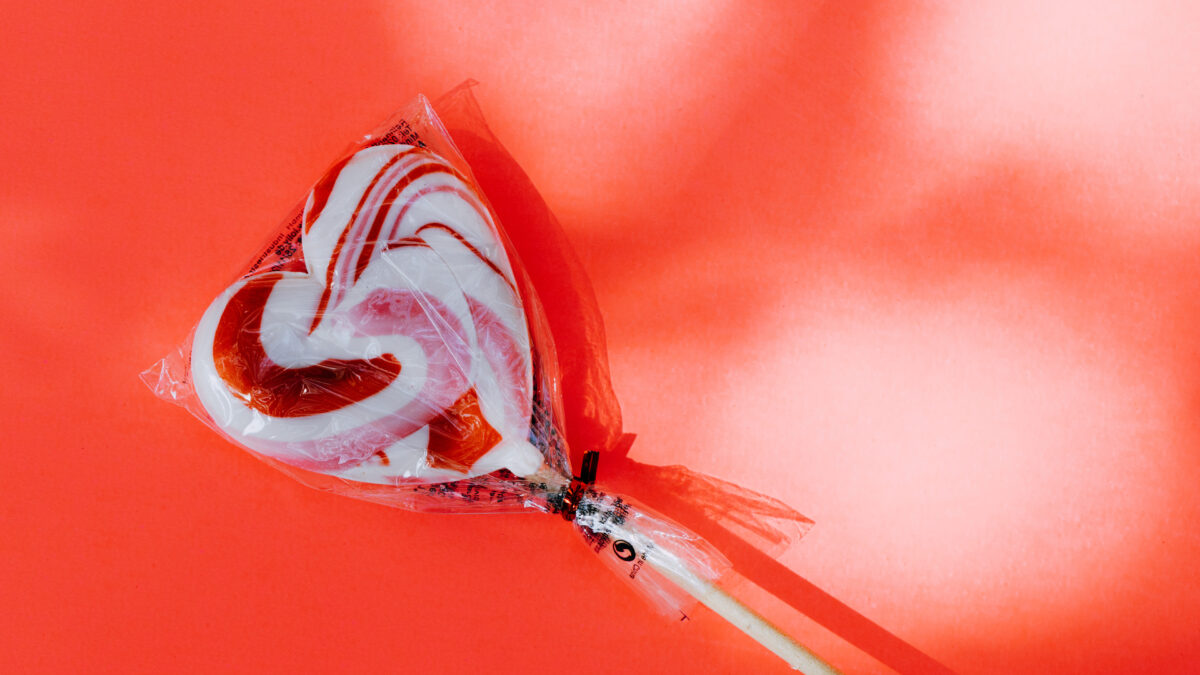
noyb vs. OpenAI: Lie another day?
OpenAI’s ChatGPT has come under scrutiny for producing responses that may not always be factually correct, a challenge commonly referred to as ‘hallucination.’ This becomes a significant concern when the generated content involves personal data, which must be accurate according to EU law. The Austrian privacy advocacy group noyb has now lodged a formal complaint with the data protection authority, claiming that the inaccuracies potentially breach the EU’s General Data Protection Regulation (GDPR) mandates.
The unbirthday incident
The case: An Austrian public figure asked ChatGPT for his birthday and kept on getting a wrong answer. This didn’t come as a big surprise: while some data concerning him was online, his birthday was not. But being congratulated on the wrong day became increasingly bothersome.
The famous Austrian turned to OpenAI, requesting a solution to this hallucination. OpenAI responded that it was not possible to block his date of birth without also blocking other pieces of information that ChatGPT would display about him. This would be excessive in light of the public’s right of information.
The subsequent complaint against OpenAI raises some interesting questions about the interaction between statistically driven LLMs and fact-driven legal requirements. Here are some initial reflections:
Accuracy first?
noyb argues that OpenAI infringes the principle of data accuracy (Art. 5 (1)(d) GDPR), which imposes an obligation to rectify inaccurate data – including birthdays invented by an algorithm. However, the cited principle has an important restriction not addressed in the complaint: It states that “every reasonable step” must be taken to ensure that inaccurate personal data is rectified. How do we define “reasonable steps” when dealing with revolutionary statistical models that generate responses based on statistical patterns rather than absolute accuracy?
Improving the trustworthiness of AI is certainly in everyone’s interest. The ability of systems to reflect on themselves, handle conflicting information and disclose lack of knowledge to users seems to be improving rapidly. Multi-layered output filters and integrating web citations in chat answers have also been helpful. However, there remains a significant technical challenge here. And given their statistical architecture, it’s rather fascinating how often LLMs already get it right. For now, warnings such as “Look for mistakes!” placed near the chat interface are supposed to manage user expectations regarding the accuracy of answers. It could even be considered that an answer should not be deemed “inaccurate” if it represents the statistically correct outcome, provided that it is clearly communicated as such (and not as a fact) to the user. But are those warnings currently clear enough?
From a legal perspective, data accuracy has long been a cornerstone of data protection law. In a landmark 1983 decision, the German Supreme Court cautioned against personality profiles of digital twins whose accuracy might not be adequately controlled. In the 2014 “right to be forgotten” case, the European Court of Justice (ECJ) ruled that search engines must remove links to personal data from search results if false information is present. The GDPR of 2018 established the accuracy principle as an abstract, yet legally binding obligation, along with a right to rectification of inaccurate personal data and right to erasure for affected individuals (Art. 16, 17 GDPR). In 2022, the ECJ ruled that the right to freedom of expression and information can be overridden when information is inaccurate.
The application of the accuracy principle requires careful interpretation based on context and a weighing of interests – it is surely not intended to universally prohibit data models based on statistics, which can significantly benefit society. To determine what is appropriate, it will be crucial to better understand what – from a technical point of view – can be done to improve the accuracy of LLM output, in particular to “delist” specific incorrect data points from the model. We also need to distinguish between actions that the provider must initiate independently, and those actions to be taken in response to a user reporting inaccurate personal data (notice and take down). It is quite surprising that noyb’s complaint does not appear to invoke the individual right to rectification/erasure of inaccurate data, even though that is the primary concern. Finally, it will be important to consider whether the inaccuracy concerns the training data itself or “only” the calculated output, and whether the output is “only” inaccurate or if it’s also defamatory, harmful to one’s reputation or otherwise illegal.
Accountability for false information
The complaint also accuses ChatGPT of “seeming to take the view that it can simply spread false information.” However, ChatGPT is neither a social media platform nor a newspaper. It provides answers to a user, who may then go on and spread the output. Holding (only) the large language model responsible for how users utilize its output ignores an important part of the problem: AI literacy.
Nevertheless, combating the spread of false information online remains a significant challenge. The emergence of large language models introduces additional complexity. We must acknowledge that false information can now be expressed with unprecedented linguistic elegance. But that’s not only a GDPR issue, it’s a broader challenge in the digital landscape in which LLMs have a special responsibility regarding the development of shared social truths in democratic societies.
Right of access – what does ChatGPT really know about you?
noyb further argues that OpenAI is obligated to provide access to the system’s training data if it pertains to the complainant’s personal information. This argument invokes Art. 15 of the GDPR. Considering the vast size of training data pools required for large language models on the one hand, and the ECJ’s recent extensive interpretation of the right of access on the other, it will be interesting to see how authorities and courts practically handle such requests. Has the rapid pace of digital development already surpassed the rationale behind the “technology-neutral” GDPR?
The GDPR does include some possibly relevant exceptions. When data is processed for statistical purposes, the right of access can be limited if it is likely to “seriously impair” the achievement of those statistical purposes (cf. Art. 89 (2) of the GDPR, implemented, for example, in Germany’s local law, Sec. 27 BDSG). However, there has been debate about whether this exception applies when data is used to train an algorithm that itself does not directly contain such data, but its application may lead to the (re)production of personal data.
-
Thomas Britz LL.M. Associated Partner